The Real AI Readiness Test: Is Your Data Clean Enough to Matter?
This week on Revenue Rehab, Brandi Starr is joined by John Williams, a fractional CRO, and Jonathan Moss, founder of AI Business Network, who believe that “AI is useless if your customer data is a chaotic mess”—and they’re here to prove it. In this episode, they challenge the widespread assumption that companies are AI-ready just because their data is accessible, arguing that fragmented, siloed data creates “context debt” and erodes trust, retention, and revenue. From eye-opening client stories to tactical fixes, John and Jonathan reveal why senior revenue leaders must prioritize data clarity before chasing AI transformation—or risk accelerating mistakes instead of results. Is your data clean enough to matter, or are you just accelerating garbage? Listen in, debate, and decide.
Episode Type: Problem Solving
Industry analysts, consultants, and founders take a bold stance on critical revenue challenges, offering insights you won’t hear anywhere else. These episodes explore common industry challenges and potential solutions through expert insights and varied perspectives.
Bullet Points of Key Topics + Chapter Markers:
Topic #1: Clean Data is the Real Prerequisite for AI Success [00:00]
John Williams and Jonathan Moss argue that most companies are rushing into AI without first fixing fundamental data problems. They challenge the popular belief that AI alone can drive revenue impact, asserting instead, “AI can’t save your revenue engine if your data is a chaotic mess.” Brandi Starr pushes for specifics, leading to a candid debate on why data readiness—not AI adoption—is the real starting line for AI ROI.
Topic #2: The Cost of Context Debt on Revenue Teams [05:37]
Jonathan Moss introduces the concept of “context debt”—the hidden tax organizations pay when fragmented data erodes efficiency and trust. He challenges the common practice of making decisions in data silos, warning that “strategic decisions on impartial information” will hurt revenue and customer relationships. Brandi spotlights Moss’s point that context debt directly leads to lost deals and missed growth, stirring debate on how leaders should audit and connect data before deploying AI.
Topic #3: RevOps, Not IT, Should Orchestrate Data Readiness [22:17]
Jonathan Moss boldly claims that revenue operations—not IT or individual business units—should own the responsibility for stitching together customer data. He disrupts the status quo: “The go to market system, which I consider data, process and technology, should be owned by RevOps.” The discussion challenges traditional data ownership models and urges CROs/CMOs to empower RevOps to connect silos, warning that without clear ownership, AI projects will fail to deliver impact.
The Wrong Approach vs. Smarter Alternative
The Wrong Approach: “They try to think about connecting all their systems to the single truth without asking the question, what decision are we trying to make that this would help us be faster and better? And so they try to take on the entire the entire project of connecting versus thinking about what, what do we need to answer and how do we, how do we do that in a better way?” – Jonathan Moss
Why It Fails: Attempting to integrate every data system all at once often leads to overwhelming complexity, wasted resources, and solutions that don’t directly support urgent business needs. Without clarity on the specific decisions the business wants to improve, massive integration projects lack focus and can stall or fail, burdening teams instead of accelerating outcomes.
The Smarter Alternative: Start with the decisions that matter most—determine which questions need answering to drive business impact and work backward to connect only the necessary data sources for those outcomes. By aligning data efforts with clear, actionable objectives, companies can deliver value quickly and ensure their data strategy fuels smarter, faster decision-making.
The Rapid-Fire Round
-
Finish this sentence: If your company has this problem, the first thing you should do is _ “List all your customer data locations. Don’t worry about whether it’s clean yet—just knowing where all your data is and what it contains is your starting point.” – John Williams
-
What’s one red flag that signals a company has this problem—but might not realize it yet? “Confusing data infrastructure with decision infrastructure. Just because your data is ‘clean’ and stored in the right place doesn’t mean it’s actionable or aligned for decision-making.” – Jonathan Moss
-
What’s the most common mistake people make when trying to fix this? “Trying to connect every system to a single source of truth without first asking what business decision you’re actually trying to make. Instead, start with the question you need to answer, then connect only the data necessary for that.” – Jonathan Moss
-
What’s the fastest action someone can take today to make progress? “Gather all your department heads for a 55-minute whiteboard session. Map out the customer journey, pinpoint each team’s touchpoints, and identify what data you have at each stage. This quickly reveals gaps and opportunities for data flow improvement.” – John Williams
Links: John Williams
-
LinkedIn: https://www.linkedin.com/in/growthcro
-
Business: https://www.sunbusinessgroup.com/
Links: Jonathan Moss
-
Business: http://www.aibusinessnetwork.ai/
Subscribe, listen, and rate/review Revenue Rehab Podcast on Apple Podcasts , Spotify , Google Podcasts , Amazon Music , or iHeart Radio and find more episodes on our website RevenueRehab.live
Brandi Starr [00:00:35]:
Welcome to another episode of Revenue Rehab. I am your host, Brandi Starr and we have another amazing episode for you today. So everybody's chasing AI, but here's the brutal truth. AI can't save your revenue engine if your data is a chaotic mess. And so today's episode we are asking the, the uncomfortable question that no one wants to face, which is your customer data clean enough to matter? Because without clarity, AI doesn't accelerate at results. We know garbage in, garbage out. It accelerates at mistakes. So we'll unpack why.
Brandi Starr [00:01:16]:
Stitching together siloed scattered data is the real starting line for AI success. We're going to why and why. Skipping the steps. Risk, wasted effort, eroded trust and loss risk Revenue. So ready for the real test of AI readiness? Let's dive in. So today I am excited that I am joined by two phenomenal guests that are powerhouse revenue leaders pushing the boundaries of AI and go to market. First, John Williams, a fractional CRO helping startups and scaleups accelerate growth through AI driven automation. And second, Jonathan Moss, founder of the AI Business Network, a master at engineering intelligent systems that scale companies from Pre seed to Fortune 500.
Brandi Starr [00:02:09]:
Gentlemen, welcome to Revenue Rehab. Your session begins now.
John Williams [00:02:16]:
Very good. Glad to be here.
Brandi Starr [00:02:19]:
I am excited to have you both and so much so we are going to just throw out the rules and skip buzzword banishment and we are going to dive straight in because you know, AI is everything right now. You can't have any conversation with anyone at this point without AI coming up. And one of the things in the race for everyone to try to adopt AI as quickly as possible and is most organizations are not really stepping back and figuring out are they actually ready for AI. And you all wrote an amazing executive brief that resonated with me so much that I had to bring you guys to the couch so that we can really dive into this. So thank you so much for joining me and for writing such a, you know, insightful, like it's short, only five or six pages, but you guys really hit on some key things and things that organizations need to think about.
Jonathan Moss [00:03:28]:
Thanks for, thanks for bringing us on. Yeah, it was a, it was a great project to work on together because it's such a, it's such a challenge that many face today. So thanks for inviting us to the couch.
Brandi Starr [00:03:38]:
Awesome. Well, I will start with the first question. What's the most dangerous assumption companies make about data when they start pursuing AI?
John Williams [00:03:51]:
So one of the things that we found is that the assumptions are that we know where all of the data is and that we're able to draw insights from the data across the organization. In practice, that ends up being that there are a few islands of heavily used information, and most of those specifically are around either the marketing ops stack or the sales ops stack, you know, in the CRM, and then the exchange of campaign information back and forth. But it seems like it falls off pretty quickly after that, even though there's a lot of really good information on the onboarding and support side as well as over on the finance side where we're talking about, you know, things like usage and billing and, and this kind of thing. So those are some of the things that I notice.
Jonathan Moss [00:04:50]:
Yeah. And I'll just add to that. And I think, I think what, what ends up happening, the problems that you end up, up causing is what we called in the, in the paper, context debt. And so you can have, you know, if you have silo data or you have data in these pockets, it's all, all not connected, then basically what you're doing is you're causing yourself to make decisions, sometimes very strategic decisions, on impartial information because you don't have all of the relevant context. And so that decision can actually end up causing downstream impacts, or vice versa, upstream impacts that you're unaware of because all of the silos aren't connected and you're not using them in the right way. So it's very dangerous to not have them connected in such a way to get all the information you need.
Brandi Starr [00:05:37]:
Yeah. And context debt, in my opinion, is one of the most powerful concepts in the executive brief that you wrote. And so for those that haven't read it yet, context debt is the invisible tax companies pay when fragmented data leads to inefficiency, lost deals, and erodes trust like that was. I'm still old school. I print things. That was one of the things that I highlighted. And so, Jay, I'd like to ask you, what is context debt look like inside of revenue teams and how can leaders spot it before it derails growth?
Jonathan Moss [00:06:15]:
Yeah. So I think, you know, you, if you, if you think about your journey, I always like to say, you know, and we'll probably talk a little about this too in a bit, is mapping out your journey and the friction points there. And what are the, what are the friction, you know, what context that is potentially causing those friction points? So let's, let's give an example. So have you ever been, have you ever been on a website and you fill out a form which is, you know, not a great buyer experience already. You wait on a meeting, you have the person who you meet with ask you similar questions that you've probably already answered or that are readily available to then get passed to someone else and then get asked those same questions again. Right. And so it's kind of like these things that you can very quickly erode trust and just on the buyer side by simply not having the all of the data in a place with that context that's being fed to each individual person that's going to have a conversation. So that's kind of a simple one.
Jonathan Moss [00:07:16]:
A more, more strategic one or more business impactful one could be the fact that, you know, you're driving a lot of, you know, business or leads with a certain type of ICP or Persona or certain type of business to then only find out that it's your highest churn. Right. And so you're doing all this work. But if you're not connecting those two things to really dive deep and understand that that is what is churning and it is an ICP Persona problem issue or a, you know, pain point or problem that you're not solving with your product, then you could continue to invest money to only lose it later on. And I think those are know from a tactical standpoint all the way up to strategic. That's kind of examples of context that.
Brandi Starr [00:07:59]:
Yeah, and I've definitely seen, you know, being a consultant, I get to see how things work inside of a lot of different organizations. And you know, bringing light to some of that context is one of the things that we've done. And a great example of that that I share often is we had a client that had a ton of content syndication vendors. I think there might have been five, seven, how many were ever were in there? And they were like, we got less money this year, we need to re reduce the number of vendors. Let's pick, you know, top two. And they looked at cost per lead and number of leads driven. So how many contacts net new contacts did they drive? And based on that data they had chose, let's say A and B. And we were like, there's not enough context there.
Brandi Starr [00:08:56]:
Like the what next? Like how many of those not even turn into business? How many of those engage with you beyond that initial download? And so we then connected that Data with what happened in their nurture campaigns. And we found that the top number one was total trash. Like literally nobody that came from that source ever did a single thing with them again. So like, yeah, you're getting a high volume at a low cost, but you're basically buying low cost trash. And there was one that had one of the higher CPLs and the volume was, you know, meh. But when we looked those people stayed highly engaged and you know, we, the way their systems work, we couldn't totally track it all the way to revenue because there was some other data issues. But even just understanding that it's like they were about to throw away their most viable lead source without that context at. John, is there anything you wanted to add to that?
John Williams [00:10:10]:
Sure. So I think that's a, that's an extremely good example of being able to just have the data and look at it within context. And again, you know, it's walk run. So in the walking stage, we're really just saying let's look at the data that we do have that's easy to get to. And then functionally. And when I say that, I mean marketing, sales. And we talked about onboarding and to a degree also product is what, what, what is our experience with the customer from a data perspective in these individual functions, how well are we creating the awareness and doing a good job of attracting that curiosity and then moving it from curiosity into a contract, you know, because there's perceived value. And then are we actually affirming that value is created for our customer, meaning onboarding.
John Williams [00:11:04]:
And so when we have these heads of functions that are communicating at least at a layer of here's the experiences that we've had this month, this week, you know, with this cohort of customers coming through that enables and empowers all parts of the GTM engine, meaning that onboarding doesn't get surprised by some different types of customers that are coming in. Marketing is not surprised by the feedback that, you know, three months later, onboarding's telling us that we're getting a lot of early churn because we're not able to establish that value quickly, even though that was in our messaging. Right. And so it's. Or different customers happen. I mean, really, seriously, let's look at the past 90 days, look at all the changes just in the narrow announcements of AI capability in the past 90 days. How has that impacted customer behavior? And so what I'm trying to get at is that if we look all the way back to the beginning of 2025, customer behavior may be Changing already from January. My goodness, you know, that was five months ago.
John Williams [00:12:17]:
So the point is, is that this live feedback loop, you know, is not a one and done. We don't do it two times a year and call it a day. This is something that functionally the teams communicate with each other because the customer's behavior is changing. And that is in the form of data right across the org. So if we're able to have that conversation about the baseline data, that allows marketing to tune messaging and make it relevant and that allows sales to have expectations of what value perception is and can we deliver that. And then that also enables the onboarding team to meet that value as quickly as possible. Right. So we can establish the value and get to recurring value quickly.
John Williams [00:13:04]:
And then product is in the backseat going, wow, some things have changed since January. This is a little more of a priority. Let's move that up on the roadmap. So I know we spent a lot of time there, but there's just some really good examples across that spectrum of how you can apply that in your GTM engine.
Brandi Starr [00:13:20]:
Yeah, and I think that's so important because so often we talk about, oh, you gotta fix data or you know, dirty data is bad, but it doesn't always send sink in as to what the real business impact is. And so talking, you know, staying with that theme in the executive brief, you also talk about five concrete business impacts of fixing data. Customer retention, profitability, product revenue, operational cost savings, and project completion rates. And so I think you both have touched on some examples already, but I would love to hear you hit it on a bit of customer retention. And but when you get into like operational cost and project completion rates, this is a place that I'd love to hear you all provide some additional concrete business impacts on fixing the data readiness. Because cost and actually getting shit done is, you know, real important.
Jonathan Moss [00:14:26]:
Yeah, so I'll, I'll start. So, yeah, so I think the, the big thing here is understanding what it is you're trying, what the objective is and what you're trying to accomplish. And I think sometimes people start with the data or start with the connecting it or cleaning it, but they, they don't. Instead of starting with what is it that I'm actually trying to solve and work my way back, what is that causing? So if I've got a scenario where I'm having extra costs because ultimately, you know, my, in my onboarding, so I'll use onboarding as an example. My ARM barding is taking too much time, which is driving up my cost Because I'm having to put more people at, at it because ultimately I don't have all the data that maps back and forth between my systems, my people and then the customer. And that's, that's very succinct and it's very, and it's in one place I'm passing spreadsheets back and forth or I'm having, I have siloed data that I have to go have someone outside of that group go find. And so thinking about it from the problem I'm trying to solve and the jobs to be done, I think is, is the key because, because if you think about data that is always going to be messy. You're never going to, you know, there's not a world I don't think we, we live in to where every, every piece of data that I'm ever going to have in my business is going to be clean and in such a way that it's like, yeah, pristine.
Jonathan Moss [00:15:52]:
Because that's going to take too much time and effort. And so really it's focus on the jobs to be done, the outcome that you're looking for and then ultimately you can surgically take on what data do I need, how do I connect my silos and then how do I use AI to be an amplifier of not only efficiency but also effectiveness in that process? And I think that's, that's the way that we would approach it. And then I can take cost out of the business because ultimately I can have less people focusing on the data transfer and more people going back to John's point, which is focusing on ensuring that my customer gets the impact that we promised them and the value that our product or our service is supposed to deliver. And that's, that's better suited for them to, for us to focus them on that because ultimately that's going to keep our customer and reduce, you know, reduce cost overall because I don't need as many people to do that data transfer and process, if that makes sense.
Brandi Starr [00:16:49]:
John, anything you want to add?
John Williams [00:16:50]:
Yeah, I've got two practical examples. So in a client we had last year, there was a pile up between sales, you know, pushing to close and hit revenue goals, doing a great job of getting customers signed up. And then just this giant bulge of onboarding is taking place across, you know, a fairly small onboarding team. Right. And so we basically have this high and a low of the customer's excited, they're ready to get going. And then, you know, here we are, you know, running into our first impressions or that, you know, it's just taking a while to get onboarded. And so one of the things that we were able to do that the data was able to help us signal upstream was to say, here are the planned projects over the next 60 days. So this is our onboarding schedule and here's our capacity.
John Williams [00:17:43]:
And Basically we have 40% capacity to sell three weeks from now. Right. And so this enabled the sales team to set expectations with customers to, you know, so that it wasn't this cold shower shock, you know, of it's going to take this long. And we ended up improving the win rate as a result because the customers who ultimately had their own timeline, they were trying to meet. Right, right. Once they understood that in order for them to meet their timeline, they actually needed to be in that on boarding cohort three weeks from now, of which there was only 40% capacity available at this moment. And so it actually accelerated the contracting process where they're like, yeah, I want to lock in because I need to be onboarded during that week. And so even that simple exchange between the onboarding team and the sales team to set expectations actually produced more than just the removing the friction out of the system.
John Williams [00:18:44]:
It, it helped in two different fronts. And so you don't have to get complicated. You just have to do, as Jay mentioned, is, you know, just think about what is it that we're trying to do. We're trying to prevent this pile up that occurs after a great successful close of the month or the quarter and then second to that on project completion rates. So, you know, a lot of times organizations are looking back into product and saying, hey, my customers are asking for this or we need this feature or this has been surfaced in responses that we're getting both from marketing and sales teams and being able to leverage AI to accelerate that product delivery, meaning that, you know, the week in and week out list of tasks of things to do just gets compressed. Okay. That time gets compressed down. And what that really means is instead of us producing two iterations of a product, you know, every 12 to 14 months, we can really bring that down into, you know, a four to six month timeline.
John Williams [00:19:47]:
So the, the chief financial officer sees that as this is, you know, booking potential. Because if we have a product to sell six months earlier, we get the advantage of selling it over those six months versus that being a sunk time cost. So I hope that's helpful, you know, hold us accountable for answering the question.
Jonathan Moss [00:20:08]:
Well, one thing and one thing, sorry, one thing I'll add too is is it's also, and we call this Out. But I don't think we called it out in, in our, in our answers. But it's also preventing future costs. And so let me give you an example. So what, what I just mentioned is what John also talked about was the capacity issue. So you got a capacity issue. What sometimes you have to do, you have to hire people to offload it. If you've got, if you've got a lot of, you know, got a lot of people onboarding or on the support side.
Jonathan Moss [00:20:36]:
If I'm growing my customer base, if I don't have some of these, you know, if I, if I don't have AI, if I don't have the data and it's taking my support team a ton of time to research tickets, figure out how to solve tickets and things of that nature. What happens is the more customers I add, the more people I have to add to support. Well, in the world where you connect your data and you connect, you know, you have AI and you have this way you can reduce the time that it takes which opens that capacity and you're actually saving future costs as you scale. And I think that's an important note to add to this as well. It's not just cost today, but it's also future costs as well.
Brandi Starr [00:21:10]:
Yeah, I love that as well. And I definitely love the onboarding example because that becomes such a win win. Because so often salespeople are trying to create a sense of urgency and most of the time it's a fake sense of urgency. Whereas that is a way to leverage your data to be really transparent with the client and create that urgency of like, I'm not trying to push you to sign for the sake of signing. If this is your timeline, here's where we need to be. If that timeline's not important, then, you know, we can onboard you at this other time. It like, it puts that control in their hands and, and sets an expectation because you may have some customers go, oh, actually if we don't start till a month from now, that is better for us because, you know, Jane is getting married and she's going to be out. You know, there's all those things that we don't know and it, it creates that partnership with your clients in a very different way when we've got that data.
Brandi Starr [00:22:17]:
And so let's talk about ownership because this is one of those things that talking to people, you know, I will talk to CMOs and they're like, yeah, I get it, our data's a mess and I could do so much more if it was better but not my monkeys, not my circus. And so where do you guys see that responsibility? So who owns the responsibility of stuff, stitching the data together? And why is that often unclear or overlooked?
Jonathan Moss [00:22:51]:
So I, I, I approach it this way. I think everyone is a steward of their own data, right? And I think they have to be owners of the data that they, you know, have direct responsibility. And, and the reason is, is because ultimately they know the most about the data. They know, they typically know that the tech stack that's giving the data they have the business context and stuff like that. So for a marketer, you know, if I'm thinking about my, the channels, if I'm thinking about the leads, I'm thinking about Personas and ICPs, like, I've got, like those are the things that I'm focused on, you know, my ads, my PPCs, my CPLs, you know, I'm giving you a bunch of lingos or paper clickers or cost per lead for the marketers out there. Well, actually in CRO's too, you should know that as well. But, but ultimately you're a steward of your data, I think, number one, and you, you should, you should own it. I think the connection piece though is an interesting question because in my mind that should be the Rev Ops team's responsibility and, and the way that we've, you know, the way that I've, I've led Rev Ops team, I lead a Rev Ops team now.
Jonathan Moss [00:23:53]:
And to me, the go to market system, which I consider data, process and technology, it should be owned by RevOps because ultimately they, they are looking at the whole entire journey from end to end and they're not siloed and their job is everyone else together as a go to market team to kind of create these mechanisms and these feedback loops that we're talking about. So there is no context, debt, et cetera. So in my mind, the owner of the data itself, you've got to be a steward of it depending upon what function you're in and the connection piece to ensure that it's flowing properly and it's, you know, as clean as it needs to be across the whole journey to me is all is Rev Ops. Now you may need some data help like data engineering and other help if it gets too complex, but ultimately to me, those are the two responsible parties, you know, in this, in this conversation.
Brandi Starr [00:24:45]:
Okay, and where do most companies overestimate their readiness in terms of AI and what signals should tell them that they're not actually prepared?
John Williams [00:25:01]:
So I would say a big signal would be how quickly can you get an accurate answer to an operational question? And that question may be something to the effect of what was our best performing set of customers in the past six months? And were they the same types of customers in the preceding six months? So we're talking about an aggregate over of a year. Right. And so the question's really about has our customer type changed in, in the trailing six months and to, you know, not get an answer on that, you know, reasonably quickly. And let's be realistic here, let's say that by next week we're going to present that information back to the executive team and accurately. Right. I think that that would indicate a red flag because even with a week's worth of work, that would be a scra companies, Right. To be able to answer a directional question such as that. Right.
John Williams [00:26:13]:
And so I think that that might indicate, hey, we, there probably is a need here and you know, Jay touched on it, that a revenue operations team is really a function like finance, legal talent, marketing. Okay. And it doesn't have to be as large as that, especially today, but we do need to have someone there to put the connecting wires together, if you will. And I think that it's that, that relates to this red flag of if your heads of functions are busy performing their functional work and they just simply lack the calendar time to go perform these operational tasks like that, okay, then that would be a red flag for saying, you know, we really need to promote this function within the organization. And that could be internally, it can also be externally. It's not like you have to do this inside. There's a wealth of resources outside of your organization who can come in and perform this task. And oftentimes that's a great option because they know what they're doing, they know what they're looking for, and they're well versed in how to put it together.
John Williams [00:27:27]:
And so those are some options for resources if you do have a red flag.
Brandi Starr [00:27:34]:
Yeah. And it's funny, like something I almost never do, but Shameless plug. That's actually what we do. It's so funny. So many people listen to Revenue Rehab and they're like, you know, I don't actually know what y' all do because generally I'm trying to share the learnings. But that, that is exactly why we exist. Because talking to, you know, we started purely as technology consultants way back 10 years ago. And what surfaced as the bigger need, I mean, people still need system admins and things for their tech, but what surfaced as the bigger need was exactly what you're Saying you've got executives, heads of functions who are running the function and running fast and doing all the things that their function is responsible for and they can't answer those simple business questions without it becoming a project.
Brandi Starr [00:28:30]:
You know, not just, oh, this is a task for next week of like, let's put this on our Kanban, let's source some resources. And it's like by that point, by the time you get an answer, everything's changed or you've missed so much opportunity. So that orchestration, and I'm really glad you hit on that, whether it's internal or external, like that orchestration is the piece that I think so many organizations are missing and undervalue, like just ridiculously.
Jonathan Moss [00:29:04]:
Yeah. And I think this goes back to the point you make is like if people are going and they're running their functions and they're making these decisions, it goes back to the point they should be stewards of their data and understand what's driving the decision that they're about to make. And is it full, you know, is it impartial or do you have all the information because you want to be able to make. You don't want to also be in this decision, you know, paralysis where you can't make a decision because you don't have everything because you need to be quick. But you also want to make sure that the decision that you're making is the most well informed with the data helping drive, you know, drive that decision or at least drive the foundation of what the decision is being made on.
Brandi Starr [00:29:46]:
So yeah, I think you, you've hit on this point a couple times in the conversation of, you know, we're not looking for perfect. And one place that I do think AI is going to help is, you know, being able to give us more complete pictures because it can aggregate and analyze, you know, so much faster and so much deeper than a human. But it is like the starting point is let's do better than the basics. Like let's not just look at data in a one dimensional fashion. Like, let's at least add some layers to make an informed decision even before we can get to those really robust. Looking at all the factors you talked about, integrating customer systems, finance systems. It's like when we've got all that data together, that is like, that's that. Oh, like where, you know, the magic really happens.
Brandi Starr [00:30:46]:
But it's like we gotta move in that direction first in order to be good enough to have the opportunity to get there.
Jonathan Moss [00:30:56]:
Yeah. And I think what AI really helps with as well is Even if, because a lot of times people also get stuck in waiting on the integration to happen or getting on someone's roadmap to get that figured out. And what I, what I always also say is, you know, especially now with AI, you have like your own data analyst at your fingertips. And so even if you can export the data to an Excel file or a CSV file or something from multiple sources or whatever, systems that aren't connected, and then put all of those spreadsheets into a chat and then start having AI help analyze it or connect dots and put things together, I mean it's such a powerful tool to be able to do those things even when you're waiting on systems to be interconnected. And I think that's, that's something that I see a lot of people don't really think about it that way or haven't approached it in such way. And I think it's just such a powerful tool. I'll probably use the data analysis capability multiple times a day for just, you know, it's cohort analysis or I've got all this data like tell me what are you, what are you connecting the dots on? Or let me ask questions of it. So I think it's very powerful.
Brandi Starr [00:32:03]:
So let me ask a little bit deeper of a question because I know you've got, you know, way more experience than myself in terms of AI. One of the things that comes up with that, because I've heard multiple people talk about this and I can say that I have not really dipped my toe in that area of AI yet. And the biggest reason why is I'm a bit unsure of what risk I'm putting my organization at by putting that information in like I know some orgs run a local, you know, large language model and that's super protected. But a lot of us are still, you know, so early on in AI usage that we don't have that in place. How can we lean into, I mean, other than the obvious removing PII like that, you know, that's pretty self explanatory, but how can we start to leverage AI in that way and minimize the risk of what we're putting out there about our organizations?
John Williams [00:33:16]:
I would definitely check the terms and conditions of whatever your favorite generative AI tool is because the answer to your information security question concerns is present in there. So for example, if you're using Google Gemini and you are a Google Enterprise customer, you have a different data privacy agreement than someone who's using, you know, the free version of Google and, and Gemini, right? And that holds true across other products. Anthropic Microsoft Xiai again, it comes back to your terms and conditions of your user agreement, generally speaking and verify this. But if you do have an enterprise subscription with one of these very large providers, my experience has been that your data privacy folds under the larger enterprise data privacy agreement. And so in the same reason that Google's not interested in reading your corporate emails, you know, to send you ads, whereas they may be interested in doing that for your personal account, you're protected in that way. So definitely take a look at your terms and conditions there. And I thought you did a great job of setting that up for, you know, changing out the pii. Right.
John Williams [00:34:44]:
So even if you don't want to use your customers company names, you know that can be Acme Corp. 1 through 257. Right. And, and these types of things. So with today's data manipulation tools, Jay covered that just now in Excel. It's, it's very easy to go ahead and just, you know, change out personally identifiable information in your original data set and then just change that. You know, if it's even private product related, it can be apples, bananas and oranges. At this point you'll just need to keep up with what's an apple and what's an orange.
John Williams [00:35:22]:
The entire point would be that, you know, if that information were released in the wild, it really would not be advantageous for someone else just other than there's some analysis of fruit that's been done on the Internet. So I love the way that you position both of those things because I do think an on site, you know, generative AI model is a little more of a complex starting out of the gate solution than being able to leverage these tools that are immediately at hand.
Jonathan Moss [00:35:55]:
Yeah, and I'll say the companies have come a long way since you know, 2022, 2023 on the, a lot of these privacies. And to John's point, you know, you, if you have teams, accounts or enterprise, you can, you typically know that your data is safe by the terms and conditions. And even on the consumer side, even if you have your own own personal information, our own personal account, you can, you actually have now more control where you can turn things on and off. Do you want it to store your data? Do you want it to, do you want it to train the model on your data? You know, they even have temporary chats. If you want to go even extra, don't train it on a model. Plus a temporary chat that go goes immediately away once I'm done with it. So they're definitely aware of this. And I think you're right to have this question because it's been one for, you know, ever since the conception of it.
Jonathan Moss [00:36:43]:
And I think that, you know, what John mentioned and those types of things are, I think they put a lot of safeguards in place now to help help kind of get over this.
Brandi Starr [00:36:51]:
Okay, and so my last question, and you guys have talked about crawl and walk a bit throughout the, the conversation. And so if a company wants to move from crawl to walk, walk to run with AI, what should they realistically be able to expect? Like it, you know what I mean? We know the, you know, the fly is when you got, you know, all the robots doing all the effective things. But like, for those people, because we got a lot of organizations that are, you know, they're barely crawling at this point. So help to paint the picture about what those different phases look like, especially related to data.
Jonathan Moss [00:37:34]:
Yeah, I would say I'll do the combination of data and AI and then, and then John, if you want to, you want to add on to that. So I think the, the crawl phase really is what we kind of call, what I would call your kind of analyst. You have, you have an intern with you. And so this intern, you are training it, you're, you're using AI to basically have, you know, certain data sets, maybe they're simplified data sets or things of that nature. Again I mentioned, you can export it from Excel or it's a couple of systems combined or things of that nature. It's really just interpreting the data, getting context from the data and really trying to help it guide you make, make better decisions and recommendations. And so I think using that day in and day out is, is like a very good, a very good crawl starting point. The, the walks, the, the next step to walk is where you actually are connecting those silos of data.
Jonathan Moss [00:38:28]:
You're being able to now have more predictive and more, you know, proactive red flags or, or green shoots, if you will. That's actually coming up every day. And so it's making recommendations to you. It's, you know, being able to have cross, you know, all this data in, in connected, you know, in a connected way where it's taken both unstructured and structured data from a qualitative and quantitative perspective to give you really good insights about whatever it is, whether it's your customer journey or your sales pipeline or your marketing channel performance or whatever it is. And so it's really kind of actually coming to you and bringing you information and Recommendations and then the run phase, which is, which is now, you know, it's possible now if you, if you know how to build agents and things, AI agents and using these tools, which is now that I can have an AI agent actually take those recommendations or take that information and actually execute a task on my behalf. And so that could be, you know, optimize my, you know, my budget across different channels based upon the data that you're seeing in my conversion rate and market, you know, marketing, whether that's Google or Facebook or something like that. That's a, that's a very, you know, good example why I don't need, you know, if I have a budget and I want to optimize for these variables, I shouldn't need to go, then do it. I could have, you know, AI or agent do that.
Jonathan Moss [00:39:50]:
And I think those. So those are like, to me, the natural kind of progression of steps depending upon where you're at along your journey. And I would be remiss to say this, most of this is not a technological issue. Most of it is a people and process issue. It's change management, it's enablement and things like that. And what you find is not having the data or the process and the people ready to take on these is a lot of times, you know, 70, 80% of the challenge that you have.
John Williams [00:40:19]:
So.
Brandi Starr [00:40:21]:
Well, we've talked about the problem, now time to fix it. Welcome to the lightning round. So this is sort of like Family Feud. Fast answers only. Let's make sure our listeners leave with actions that they can take right now. So four questions. I will direct each to one of you and the goal is quick advice. So, number one, John, finish this sentence.
Brandi Starr [00:40:48]:
If your company wants to unlock AI value, the first thing you should audit.
John Williams [00:40:53]:
Is your data locations. So having a list of where customer data lives in the organization. Don't care if it's clean, just need to know where it is and what it is is a beginning takeaway. So having that list, you can manage what you know.
Brandi Starr [00:41:12]:
All right, Jay, next one's for you. What's one subtle red flag that signals a company's data isn't AI ready, even if leadership thinks it is.
Jonathan Moss [00:41:23]:
So I think companies confuse data infrastructure with decision infrastructure. So just because your data is clean and connected in a place, as John mentioned, doesn't mean it's actionable or aligned. And so ensuring that you have that as well is important when you put AI on top of it.
Brandi Starr [00:41:39]:
All right, I'm going to come back to you. John for number or Jay for number. Three, what's the most common mistake teams make when trying to stitch data together for AI?
Jonathan Moss [00:41:49]:
They try to think about connecting all their systems to the single truth without asking the question, what decision are we trying to make that this would help us be faster and better? And so they try to take on the entire the entire project of connecting versus thinking about what, what do we need to answer and how do we, how do we do that in a better way?
Brandi Starr [00:42:06]:
And final question for John. What's one tactical move companies can take today to improve their data clarity or flow?
John Williams [00:42:15]:
I would get the heads of functions together for a 55 minute whiteboard session. Draw an arc of the customer journey and then at each point where those functions involve, what are the main touch points of the customer? And what data do we have that can help us support support that touch point.
Brandi Starr [00:42:33]:
Awesome. I love it. Every good session ends with a plan for progress because just talking about the problem won't fix it. Well, before we go, I want to give you all the opportunity to tell the audience where they can connect with you. And I know both of you run your own businesses, so definitely give the shameless plug and tell us what you do. So, John, I'll start with you.
John Williams [00:42:58]:
Awesome. So you can find me on LinkedIn, which is where I post the most Most as growth CRO. And you will find my businesses sunbusinessgroup.com and would love to help you out if you need some help.
Brandi Starr [00:43:13]:
And Jay.
Jonathan Moss [00:43:15]:
All right, so AIBusinessNetwork.ai, you can find me on LinkedIn, mainly X as well, and YouTube. Basically what we do is we help companies or individuals, specifically leaders, on their AI journey. So our goal is to bridge the gap between AI literacy and business impact. So wherever you're at, we're kind of the resource for education and training, research frameworks, anything that you need to help you along your journey.
Brandi Starr [00:43:46]:
Awesome. Well, we will make sure to link to your socials as well as your businesses. So wherever you are listening or watching this podcast, check the show notes so that you can connect with John and Jay. Well, thank you guys so much. I have truly enjoyed this discussion. You know, data is exciting to me, even if it isn't to everybody else. So I have definitely had fun. So thank y' all.
Brandi Starr [00:44:14]:
Thank you and thanks everyone for joining us. I hope you have enjoyed our discussion. I can't believe we're at the end. Until next time, bye. Bye.
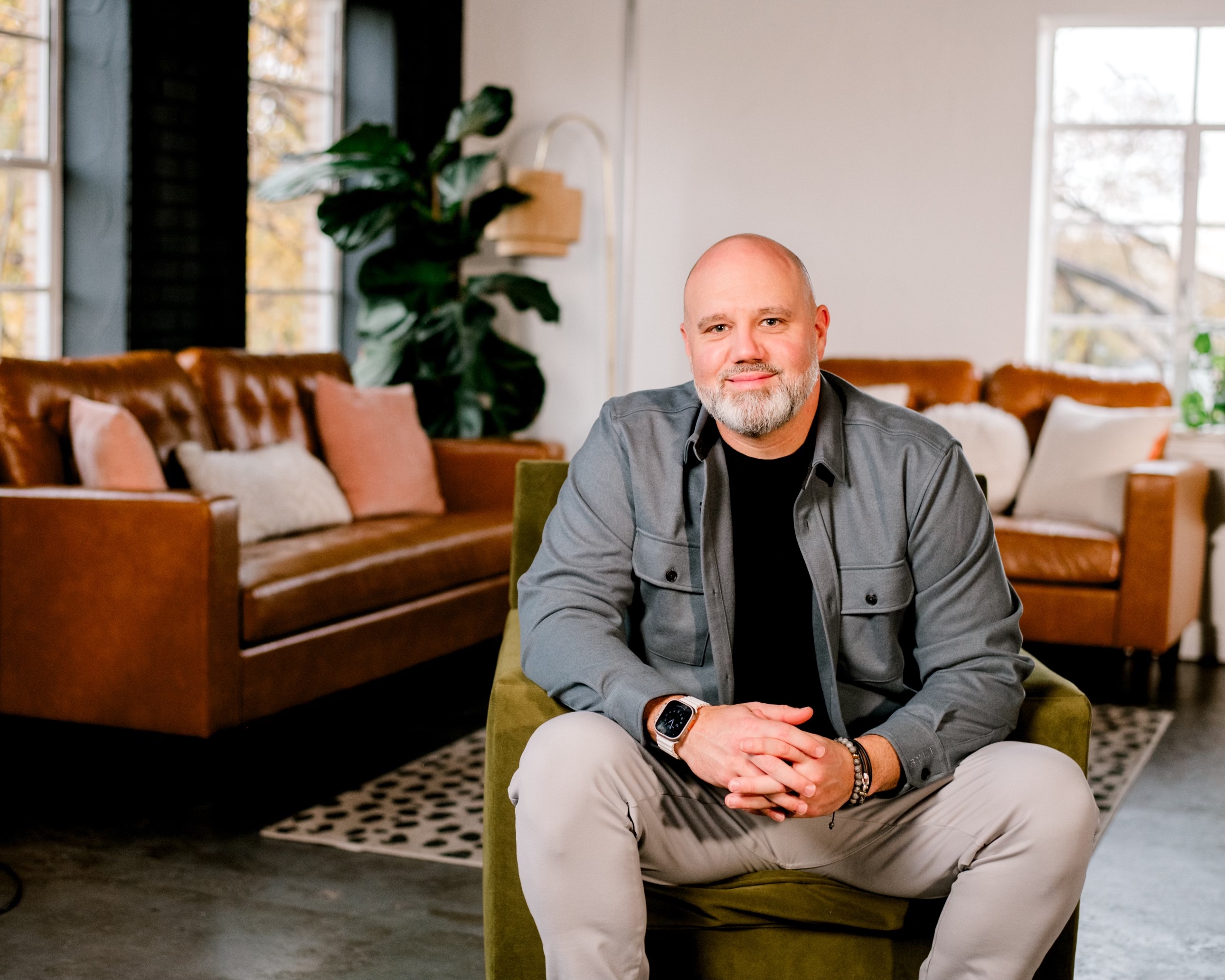
Jonathan Moss
EVP - Growth and Operations @Experity; Co-Founder @ AI Business Network
Jonathan is an executive, founder, and advisor focused on business strategy, go-to-market, and operations. He has worked for and with companies of all sizes from Fortune 500 to Pre-Seed startups. His superpower is engineering growth and building intelligent systems that leverage AI and revenue architecture. This helps companies scale and build durable businesses.
He is the Co-Chair of AI in GTM function for Pavilion and a Winning by Design Go-To-Market Ambassador.
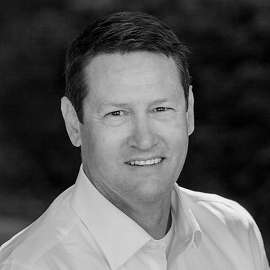
John Williams
Chief Revenue Officer (fXO)
I have spent the last 4 years as an independent solopreneur focused on helping late-stage startups and early to mid-stage scaleups execute in the growth curve challenges present in their existing environment and build for sustained performance in upcoming growth areas. These are usually around the go-to-market function encompassing marketing, sales, onboarding and support success teams, coupled with product, talent, finance, and legal.
The focus on sustainable, fiscal growth is a welcome pivot and AI capabilities provide GTM teams with efficient and effective production in the right configuration; adoption is the basis for success, and learning how to apply the advantage as individual operators is the key differentiator of successful operations.